Listen to the story below:
When first introduced to physics as a middle-school student, Aobo Li admits he found the subject boring. It was difficult, and he couldn’t connect the elementary principles taught with what he calls “the underlying interactions of nature” that fascinated him. As he grew older, curiosity led him to explore philosophy, religion, and even applied math, but eventually, Li found what he was looking for in the field of particle physics: neutrinos.
“I wanted to understand how nature works and how we interact with it,” Li admits. “I wanted to know what’s really happening. What are the underlying principles behind the things we see? We see the sky is blue, but why is it blue?”
Now, as a postdoctoral researcher in the Department of Physics and Astronomy at UNC-Chapel Hill, Li develops innovative machine learning algorithms and applies them to neutrino detectors, advancing fundamental physics research.
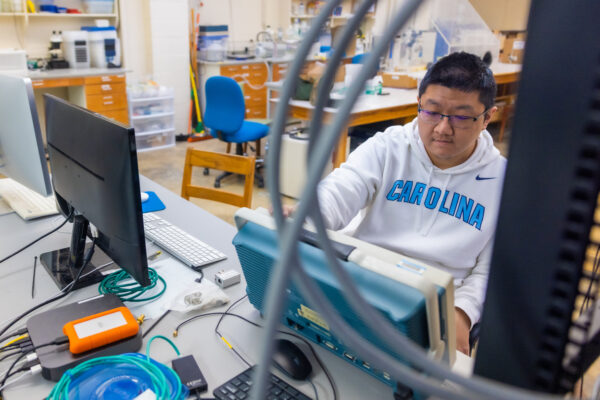
Li uses machine learning to detect neutrinos. (photo by Andrew Russell)
Neutrinos constitute the building blocks of the universe. Unlike many other subatomic particles, neutrinos are elementary, meaning they can’t be subdivided. A million times less massive than electrons, they can be created by potassium’s radioactive decay inside the human body, in nuclear reactors and particle accelerators on Earth, and by the nuclear processes inside stars. The highest-energy neutrinos come from far out in space. Scientists believe they are born in giant active galactic nuclei called blazars, powered by material falling into supermassive black holes.
“My deepest research interest is to understand what’s actually happening within nature,” Li says. “Unfortunately, nature doesn’t speak English. So, what we have to do as physicists is to convert its message into something that we can actually understand.”
Coming to America
Li grew up in Jinan in the Shandong Province of China. During his freshman year, he learned of an exchange program run by an international non-governmental organization, the Laurentian Institute, that helped Chinese students attend high school in the United States.
“My mom thought it was a great opportunity,” Li says. “In the beginning, I was slightly against it because the U.S. was so far away. But my mom insisted. She said: ‘You should go out and see the world!'”
He ended up in San Pedro, California, attending a Catholic high school, and then enrolled at the University of Washington (UW) in Seattle.
When he got to UW, he tried to pursue computer science, thinking the major would make him more employable after graduation. But the school has one of the world’s most competitive computer science programs, and Li’s GPA needed to be higher for the department. Disappointed, he was forced to consider other majors. On a whim, he enrolled in a particle physics course over the summer session of his sophomore year.
Li did well in the class, but was still unsure about a career in physics. At the end of summer, he visited an aunt in Canada, and her advice helped him commit.
“You’re only 20 years old,” she told him. “You’re still so young. Give it 10 years. If you try your hardest, and it doesn’t work, you’ll just be 30 — and you can still switch tracks.”
As an undergraduate, Li’s advisor Jason Detweiler guided his course load, helped him participate in research, and met with him for weekly advisory sessions — a rare opportunity. It was during these meetings that Li was introduced to neutrino physics.
“I think of him as kind of the shepherd of my career,” Li says. “I heard Jason keeps bragging about me, so I guess he still likes me.”
After UW, Li started working towards his PhD at Boston University (BU) with advisor Chris Grant. Most physics PhD candidates take the traditional path of adopting a topic studied by their advisor, following their guidelines to graduate. This didn’t appeal to Li. He wanted to find something unique to work on — something only he could do. He pitched this unorthodox path to Grant, who told Li that if he could find a good topic, Grant would support him.
Soon after, Grant introduced Li to Lindley Winslow, a physicist from the Massachusetts Institute of Technology (MIT). BU was collaborating with MIT and physicists from around the world in an experiment examining the nature of neutrinos called KamLAND-Zen. Winslow tapped Li to attend a five-day machine learning boot camp at MIT. There, Li began to see his PhD topic emerge.
“As things unfolded, I started connecting the dots,” he says. “We learned this model called Convolutional Neural Network, which we use to analyze an image. I realized that our detector is also producing an image. I thought, Why can’t we use this model to analyze our detector data? So, that’s when it all started.”
Learning curve
Neutrinos’ small size allows them to easily pass through matter, carrying information about their origin — information unchanged by external interactions. This makes neutrinos attractive to scientists for study, but it’s also what makes them so challenging to detect.
For every 10 trillion neutrinos passing through the planet, only one interacts with the earth, making them extremely difficult to detect in nature. In fact, the first neutrino wasn’t detected until 1956.
The common characteristic all neutrino detectors share is that each takes a neutrino event — an interaction of neutrinos — and converts it into data that displays as an image or a waveform that communicates the properties. Scientists then decode the data represented by these pictures or waveforms into actual physics parameters that describe the event.
In this decoding, Li saw an opportunity for machine learning to support his research.
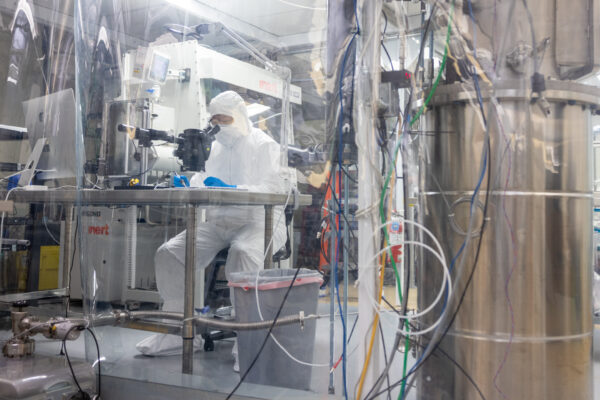
Li looks through a microscope in the Gruszko Lab. (photo by Andrew Russell)
To explain the basic principles, think of a cat. If traditional physicists were to attempt to identify a cat, they would rely upon what are known as “first principles” — predefined attributes assigned to an object. Then, they would make judgments about observable characteristics like fur, triangle-shaped ears, and whiskers.
But a problem arises when the image of the cat is incomplete. For example, the ears may not be seen, or the whiskers may be obscured. These missing attributes can skew the physicist’s judgment. This becomes particularly important when using analysis technology for neutrino detection because it’s a highly complex system, and the data captured often doesn’t align with the first principles.
With machine learning, physicists don’t need the first principles. Foregoing the idea of “cathood,” instead, they feed hundreds — sometimes thousands — of photos into a statistical model, which learns the first-principle features by itself. It can extract information from the lowest level of data, like the raw output of the image or waveform from the detector, and directly learn physical principles. Then, it converts those concrete images and waveforms into abstract physics.
Li took the basic principles he learned from the boot camp and developed a cutting-edge machine-learning model for the KamLAND-Zen experiment called KamNet. The model vastly improved the performance of the experiment — the equivalent of millions of dollars of investment.
In January of 2023, KamLAND-Zen published groundbreaking results. Li’s work on the experiment earned him his PhD and the prestigious Dissertation Award in Nuclear Physics from the American Physical Society. It also provided him with a launch pad for his postdoctoral work.
Theory of everything
After Li graduated, he received two offers for postdoctoral work: one at Columbia and the other at UNC-Chapel Hill. For Li, the two universities offered very different opportunities. Columbia represented the center of the machine learning effort in the U.S., and many of their experts were already utilizing the subfield of artificial intelligence in physics.
Like his PhD project, he wanted to do something unique. Carolina represented an opportunity to develop a project from the ground up and take on a leadership role in the department.
“I have to say, I was pretty bold back then,” Li says with a laugh. “I’m a pretty independent, self-motivated person. I was excited to come to this new unexplored territory, this uncharted land, and try to build my own thing.”
Another major factor that drew Li to UNC-Chapel Hill was the opportunity to work in the lab of Julieta Gruszko. The two first met at UW, where Gruszko was a graduate student. Among other projects, her lab works on the LEGEND experiment, a collaboration of over 250 researchers from about 50 institutions worldwide. That research team looks for a hypothetical phenomenon called neutrinoless double beta decay that, if observed, would expand what physicists know about the nature of our universe.
According to the known laws of physics, combining equal amounts of matter and antimatter will cause the two to annihilate each other, releasing an enormous amount of energy. The reverse is true: The release of any quantity of energy should convert to equal amounts of matter and antimatter.
When the Big Bang occurred, equal amounts of matter and antimatter should have been released into the universe, but the universe appears to consist of much more matter than antimatter. The neutrinoless double beta decay experiment examines the presence or absence of neutrinos in the decay of a nucleus. This theoretical interaction could provide insight into the question: Why is there something and not nothing?
Now, Li isn’t just asking questions like why the sky is blue — he’s exploring why anything exists at all.
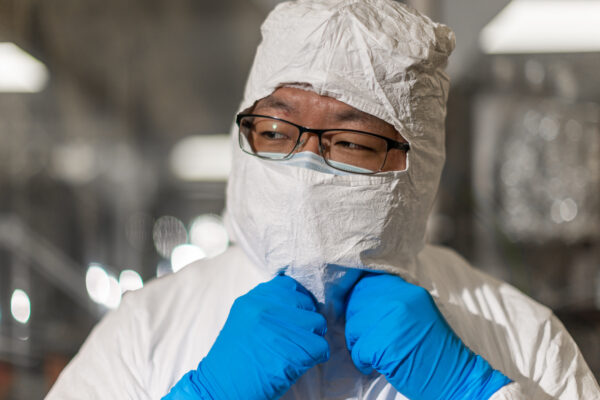
Li dresses in a “bunny suit,” which is used to protect sensitive equipment in a lab space on the UNC-Chapel Hill campus. (photo by Andrew Russell)
Li’s machine-learning model expanded the Gruszko Lab’s ability to view neutrino events. As part of his work on LEGEND, Li initiated the Germanium Machine Learning (GeM) group. It currently has two postdoctoral researchers, five graduate students, and one undergraduate student across several U.S. and E.U. institutions. Li created a training pipeline for GeM collaborators to teach them how to head their own machine-learning projects.
Li’s work at Carolina led to him winning this year’s Machine Learning: Science and Technology Paper Award from Machine Learning and the Physical Sciences Workshop at the 2022 Conference on Neural Information Processing Systems, one of the most prestigious conferences about AI and machine learning. Additionally, his work on LEGEND and KamLAND-Zen earned him a Postdoctoral Award for Research Excellence last year.
“Physics has probably some of the smartest people in the world,” Li says. “If there is one thing that I’m doing better than other people, it’s that I’m highly self-motivated and very curious about a lot of things. My research is not just physics, not just neutrinos. I’m kind of like an interdisciplinary scientist. I use artificial intelligence and machine learning to probe the data from our neutrino detector.”
Li won these awards last year, the same year he turned 30. He recalls his aunt’s advice about giving physics 10 years before deciding whether to switch tracks.
“I think I’m pretty happy with my career in physics so far,” Li says, laughing. “I love my research, I love the people I work with, and I’ve been pretty successful, so I think I will stick with it.”